Your cart is currently empty!
IBM Watson Assistant provides better intent classification than other commercial products…
IBM Watson Assistant provides better intent classification than other commercial products according to published study
And how the better performances of IBM Watson Assistant tackle the most common pain points in the adoption of a conversational solution
Introduction
Today, virtual assistants (or chatbots) represent a globally recognised booming trend:
With regards to chatbots, which are in many ways the most recognisable form of AI, 80% of sales and marketing leaders say they already use these in their CX or plan to do so by 2020¹.
25 percent of customer service operations will use virtual customer assistants by 2020².
By 2025, customer service organizations that embed AI in their multichannel customer engagement platform will elevate operational efficiency by 25%³.
It is clear that chatbots are here to stay.
Their limitless potential in terms of business versatility — is there any business that does not implement customer service operations? — , maturity of technologies, and absolute clarity of matching use cases and expectations, make the virtual assistant one of the most immediately adoptable A.I. solution in any company.

Nevertheless, if you have ever used a chatbot, you may have experienced the frustration coming from the reiterated invitations to rephrase even apparently simple questions (“Sorry, I do not understand.”), and a slight, yet non-negligible, sense of distrust of any virtual assistants’ comprehension abilities matured from firsthand experience.
In fact, it is also estimated that:
40% of chatbot/virtual assistant applications launched in 2018 will have been abandoned by 2020³.
There are several conversational technologies that could be adopted when implementing a virtual assistant. Nowadays, every major Cloud vendor provides a conversational API infused with A.I. capabilities in its service catalogs. To cite a few:
- IBM Watson Assistant⁴
- Google Dialogflow⁵
- Microsoft LUIS⁶
- Amazon Lex⁷
- Oracle Digital Assistant⁸
- . . . and more
Trending Bot Articles:
1. The Messenger Rules for European Facebook Pages Are Changing. Here’s What You Need to Know
3. Facebook acquires Kustomer: an end for chatbots businesses?
It is also possible to adopt open-source conversational services (such as RASA⁹) or even create your own by leveraging publicly available Natural Language Processing (NLP) projects, like BERT¹⁰.
This post highlights some of the advantages of IBM Watson Assistant in comparison to other commercial tools, based on the evidences reported in the recent technical paper by Qi et al. (2020)¹¹. In doing so, we will start by the most common pain points that occur with the adoption of a virtual assistant, and comment how the study findings address them.
The chatbot does not perform well in terms of understanding
Intent detection, the classification of the user’s utterance into a predefined class (or intent), is the solid pillar upon which any task-oriented conversational system is built.
An example of intent in the banking field may be “Card Lost”, as shown by Casanueva et al. (2020)¹² :

The classification of an input sentence as an intent allows the conversational service to identify the reply for the user, through an existing mapping between the intent label and a node in the conversational tree. Therefore, the intent misclassification may lead to either wrong replies or no reply at all (“Sorry, I do not understand.”). Understandably, intent’s misclassification represent the first point of failure and the nightmare of any conversational solution.
IBM Watson Assistant provides a new intent detection pipeline that leverages complex Machine Learning algorithms and methodologies — namely transfer learning, AutoML and Metalearning¹¹.
The study by Qi et al. (2020)¹¹ shows that IBM Watson Assistant outperforms other commercial solutions in terms of accuracy in the task of intent detection:
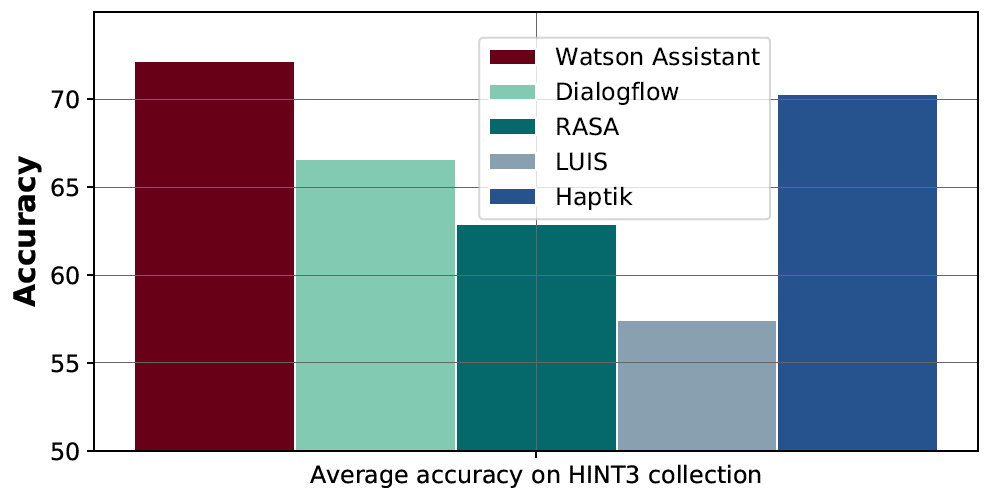
The same benchmark and testing methodology as Arora et al. (2020)¹³ were used. In particular, Arora compared Google Dialogflow⁵, Microsoft LUIS⁶, RASA⁹ and BERT¹⁰ with Haptik¹⁴. As IBM Watson Assistant was not considered in the first place, Qi et al. (2020)¹¹ used the same data sets and experimental setup to add it to the benchmark and obtain comparable results.
According to the study, IBM Watson Assistant shows higher accuracy than than Google Dialogflow (+5.6%), and Microsoft LUIS (+14.7%), on the same data set and in the same testing conditions.
How many sentences do I have to add to make the chatbot work well?
When planning for the adoption of a conversational solution, an important concern is about the effort required to train and maintain it.
Despite the presence of established approaches to the measurement of fair performances, and the availability of different performance metrics, the fear that no training data set will be “complete” enough to work reasonably on a real scenario may be blocking.
Frequent questions involve: “How many utterances do I need to train the system properly?” or “How many people should be assigned to the task of manufacturing training samples?”
Qi et al. (2020)¹¹ also tested the technologies on smaller data sets (“Subsets”), created by discarding semantically similar sentences from the original data sets (“Full”), to investigate the behaviour of the models with reduced training set size:
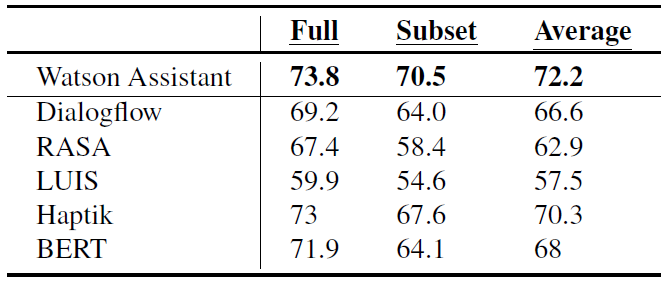
According to their study, not only IBM Watson Assistant outperforms other commercially available products, but also displays the smallest drop in accuracy when the size of the data sets decreases, possibly making it a better choice in scenarios with a minor amount of input data.
Adding new examples/intents and re-training is prohibitive
On the surface, the implementation of a chatbot may seem mainly a technological matter. But it is not.
Indeed, the adoption of a virtual assistant requires significant operational adaptations, as periodic trainings must be taken into account. Although this happens for every Machine Learning project, for chatbots — unlike in the case of labeled, clean tabular data— the definition of new intents, relevant training utterances, the segmentation of the knowledge base to manufacture suitable replies, need the infusion of specific business knowledge, the support of a Subject Matter Expert (SME) and, for most cases, is not an automatic process.
An example: a company launches a new product into the market; as a consequence, users will ask new questions concerning the product to the virtual assistant. The SMEs should be involved to identify the potential questions (they may also overlap with already existing ones) and manufacture adequate replies.
For these reasons, a company would need a conversational service:
- easy to train: user-friendliness fosters business adoption.
- that trains rapidly: quicker and simpler training sessions result in smoother enhancements of the knowledge base.
The lack of one of these conditions may result in the failure to adopt the solution and its abandonment in the long run.
Both conditions are met by IBM Watson Assistant, that provides an extremely intuitive graphical user interface that does not require technical knowledge to be effectively employed.
Qi et al. (2020)¹¹ measured the training time required by different Natural Language Understanding (NLU) engines in order to process the same input data set, and IBM Watson Assistant offered the best trade-off between final accuracy and training time:
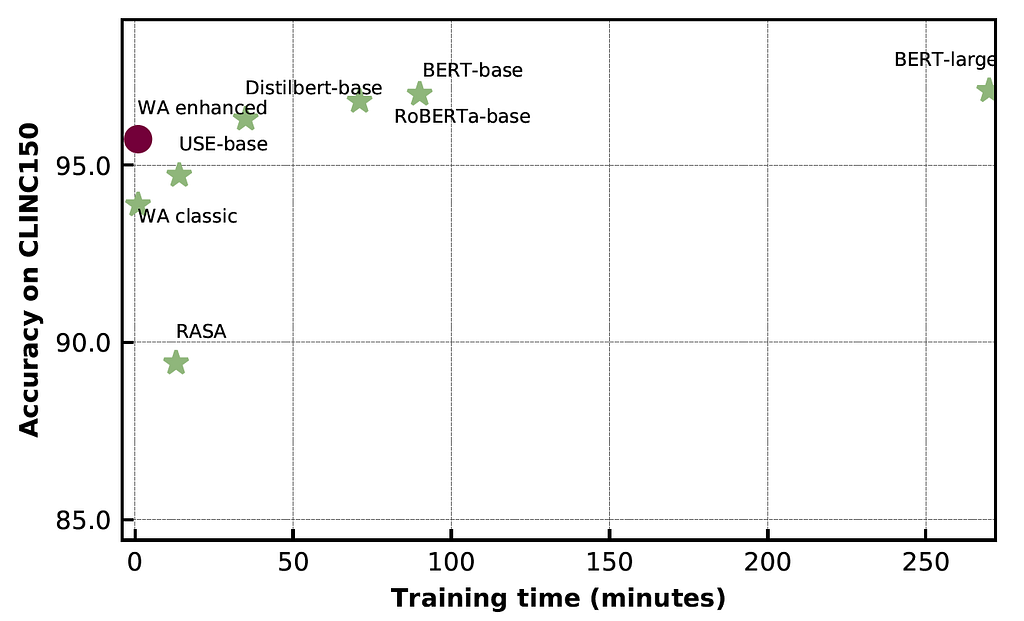
Who is going to use the tool?
The user-friendliness of the tool is not object of investigation in the cited study, but it is important to mention that, as IBM Watson Assistant is easy to use and does not require a strictly technical skillset, it encourages its adoption by business users.
Business users alone possess the specific domain knowledge and peculiar jargon to be imbued into the chatbot. Empowering them with a friendly instrument that allows easy modifications is paramount to the success of a conversational solution.
Conclusions
In this post, we commented the results of the paper from Qi et al. (2020)¹¹, which, by comparing different conversational services from multiple vendors, shows that IBM Watson Assistant displays generally better performances, in terms of:
- Higher intent detection accuracy.
- Minor drop in accuracy with less input data (smaller training sets).
- Best trade-off between accuracy and training time.
We also remarked how these results address the most common pain points that appear with the adoption of virtual assistants, together with the user-friendliness characterizing the tool.
This post does not intend to provide an exhaustive overview of the differentiating capabilities offered by IBM Watson Assistant, such as its irrelevance detection features¹⁵ or its native integration with search services¹⁶ (namely IBM Watson Discovery¹⁷) to better support navigation through large document corpora within the chat and manage questions in the “long-tail” . This goes beyond the scope of this article.
References
[1] Oracle, “Can Virtual Experiences Replace Reality?”, link.
[2] Gartner, “Gartner Says 25 Percent of Customer Service Operations Will Use Virtual Customer Assistants by 2020”, link.
[3] Gartner, “Market Guide for Virtual Customer Assistants”, link.
[4] https://www.ibm.com/cloud/watson-assistant
[5] https://cloud.google.com/dialogflow
[7] https://aws.amazon.com/lex/
[8] https://www.oracle.com/chatbots/digital-assistant-platform/
[10] Jacob Devlin, Ming-Wei Chang, Kenton Lee, Kristina Toutanova, “BERT: Pre-training of Deep Bidirectional Transformers for Language Understanding”, 2019, arXiv:1810.04805, link.
[11] Haode Qi, Lin Pan, Atin Sood, Abhishek Shah, Ladislav Kunc, Saloni Potdar, “Benchmarking Intent Detection for Task-Oriented Dialog Systems”, 2020, arXiv:2012.03929, link.
[12] Iñigo Casanueva, Tadas Temčinas, Daniela Gerz, Matthew Henderson, Ivan Vulić, “Efficient Intent Detection with Dual Sentence Encoders”, 2020, arXiv:2003.04807, link.
[13] Gaurav Arora, Chirag Jain, Manas Chaturvedi, Krupal Modi, “HINT3: Raising the bar for Intent Detection in the Wild”, 2020, link.
[15] https://medium.com/ibm-watson/enhanced-offtopic-90b2dadf0ef1
[16] https://medium.com/ibm-watson/adding-search-to-watson-assistant-99e4e81839e5
[17] https://www.ibm.com/cloud/watson-discovery
Don’t forget to give us your 👏 !
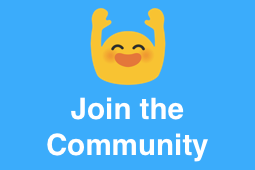
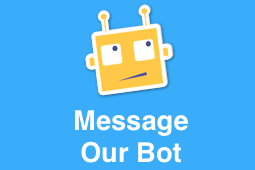
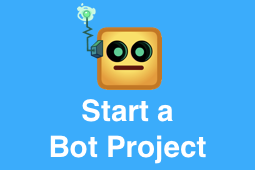
IBM Watson Assistant provides better intent classification than other commercial products… was originally published in Chatbots Life on Medium, where people are continuing the conversation by highlighting and responding to this story.